Large language models like OpenAI’s ChatGPT have emerged as game-changers in the world of artificial intelligence and natural language processing, extending their influence into fields such as marketing with innovative services. The computational power and the costs associated with training these models are substantial, and our aim is to shed light on these aspects, offering clarity and demystifying the complexities involved.
Unravelling the Computational Colossus
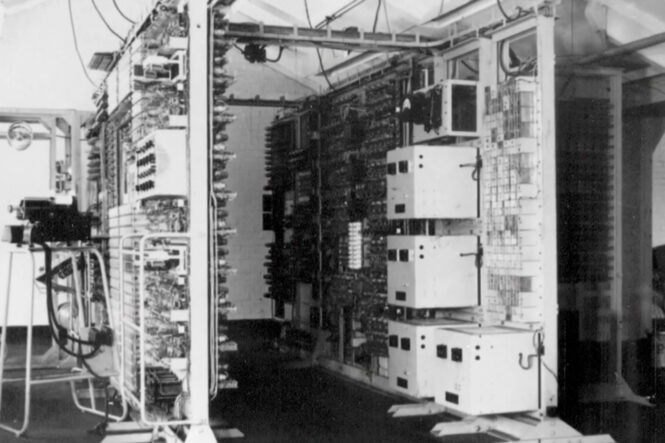
Modern language models, such as GPT-3, are colossal in their complexity, housing as many as 175 billion parameters. To facilitate learning, these models require extensive data, coupled with a vast amount of computational power to process it. Training these models is an event that, while occurring only once, can stretch over weeks, or even months, depending on the hardware utilised. Services like Generative SEO have begun to harness this power to revolutionise their domains.
The computational power required for such an operation is typically quantified in floating-point operations per second (FLOPS) — a unit that measures how many calculations a computer can perform per second. It’s astonishing to comprehend that training GPT-3 is estimated to require around 3.14 x 1023 FLOPS (source https://arxiv.org/abs/2005.14165).
Behind the Scenes: The Hardware
The training of a large language model is a process generally handled by graphics processing units (GPUs) due to their capacity to perform parallel operations and handle large volumes of data more efficiently than traditional CPUs. High-end GPUs or clusters of GPUs are often deployed for such tasks. The use of such high-performance hardware significantly contributes to the overall cost of training.
Unpacking the Costs
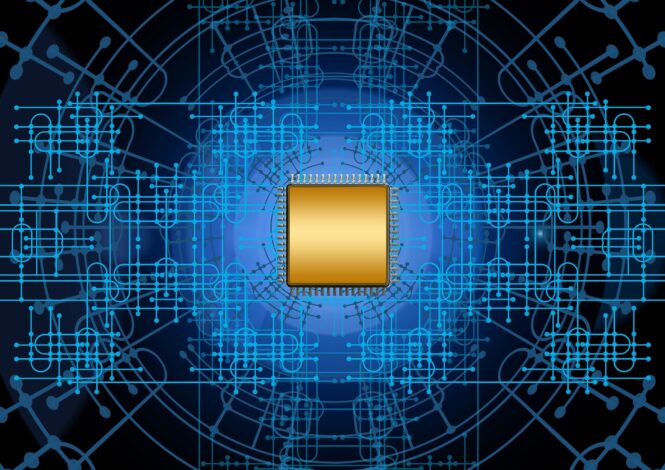
Understanding the cost components of training large language models requires consideration of factors like model complexity, hardware specifications, energy consumption, and even regional electricity costs. For the purposes of our discussion, let’s break down the approximate costs associated with training a model like GPT-3, assuming we are using commercially available cloud computing resources:
Initial Training Costs
Resource | Approximate Cost |
Computing power (GPU hours) | $4.6 – $12 million |
Energy consumption | $1,000 – $10,000 |
Storage, data, and other associated costs | Varies |
Post-Training: Day-to-Day Running Costs
Once the model has been trained, the computational requirements and associated costs drop significantly. However, to keep the model operational and maintain its efficiency, continuous costs like server maintenance, energy consumption, and software updates are inevitable. Let’s break down these ongoing costs:
Resource | Approximate Cost |
Server costs (cloud server rental) | $1,000 – $3,000 per month |
Energy consumption | $100 – $1,000 per month |
Maintenance, updates, and security | Varies |
Human Capital: An Overlooked Cost
Beyond hardware and energy expenses, the human expertise required to develop, train, and maintain these models represents a significant cost. Highly skilled data scientists, machine learning engineers, and infrastructure experts are essential for the successful development and operation of such models. The costs associated with hiring and retaining these professionals can be substantial, particularly given the high demand and relatively limited supply of such specialised skill sets.
The Energy Component: An Environmental and Economic Consideration
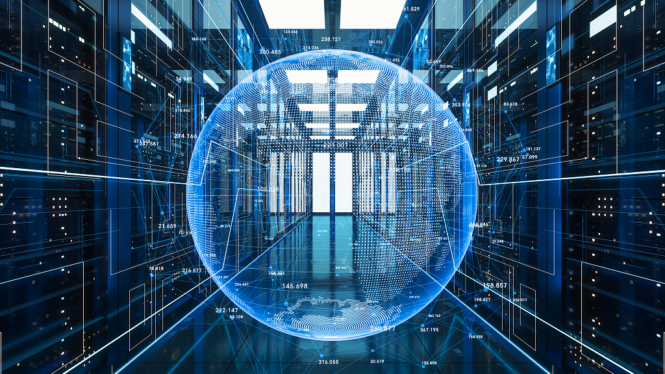
One cannot overlook the energy usage while discussing the costs and computing power associated with training large language models. In fact, the energy consumption involved in training AI models has been a subject of increasing concern from both environmental and economic perspectives. Researchers have found that training a single large AI model can generate carbon emissions equivalent to five times the lifetime emissions of an average car (source: https://arxiv.org/abs/1906.02243).
The exact energy cost can vary widely depending on the specific computational setup, the efficiency of the hardware, the model’s complexity, and the cost of electricity in a given region. However, it’s essential to consider this element due to its potential environmental impact and the additional expenses it incurs.
The Need for Redundancies: A Factor in Cost
In the vast computational endeavour that training these AI models is, redundancy plays a crucial role. These models often need to be trained multiple times to fine-tune their parameters and optimise their performance. Furthermore, backup copies of models are also essential to ensure the preservation of the considerable time and resource investment they represent. These redundancies can increase the computing power required and therefore the overall cost.
Model Maintenance and Update: A Continual Process
Training a language model like GPT-3 is not a one-off process. To remain useful and relevant, models need to be continually updated and fine-tuned. This means retraining the model with new data to reflect changes in language use, societal norms, or specific business requirements. The costs of these ongoing updates add to the total cost of ownership for large language models. This also entails a constant need for computational power, contributing to the total energy usage and associated costs.
Democratising AI: Tackling the Cost Barrier
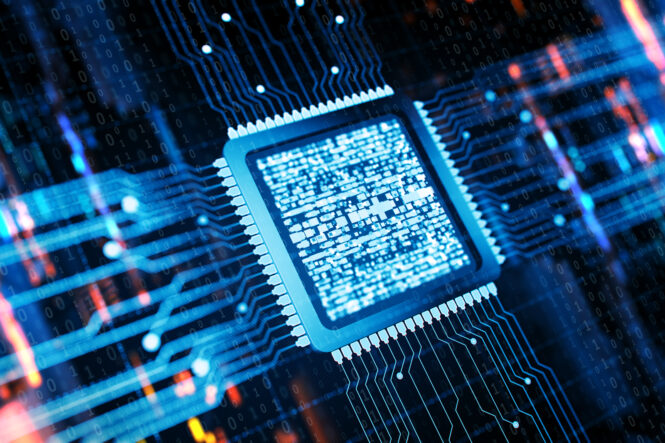
The cost of training large language models poses a barrier to entry for many smaller organisations and researchers. Recognising this, some organisations are exploring ways to make AI more accessible. Shared resources, pre-trained models, and cloud-based AI services have emerged as potential solutions to democratise access to AI. By leveraging these resources, smaller organisations and individuals can utilise AI without the enormous expense of training models from scratch.
In conclusion, the computing power and associated costs involved in training models like GPT-3 are vast, spanning hardware expenses, energy consumption, and human expertise. Nevertheless, the benefits these models can offer are substantial, offering new opportunities for automation, innovation, and efficiencies across various sectors.
Justifying the Investment: The Power of Language Models
Given the considerable costs and resources required to train and operate large language models, it’s reasonable to question their worth. The answer lies in the transformative potential of these models. From enhancing customer service interactions to improving medical diagnoses, the applications of language models like GPT-3 are wide-ranging and can create significant value. By automating complex tasks, these models can drive operational efficiencies and foster innovation, justifying their substantial cost for many organisations.
Looking Forward: Trends in Cost and Efficiency
While the current costs of training and maintaining large language models are significant, technological advancements promise greater efficiency in the future. As hardware becomes more powerful and algorithms more efficient, the computational requirements for such models are likely to decrease, making them more accessible (source: https://arxiv.org/abs/2001.08361). At the same time, cloud-based AI services are becoming increasingly popular, offering access to high-performance models without the high upfront training costs.
Conclusion
Training a large language model like ChatGPT is a resource-intensive process that requires significant computing power and associated investment. However, the potential applications and the value these models can bring, in areas ranging from customer service to medical diagnosis, content creation, and more, make this a worthwhile investment for many organisations. With the advancement of technology, the expenses and resources needed for training these models are expected to go down. This will make the models accessible to a larger group of users.